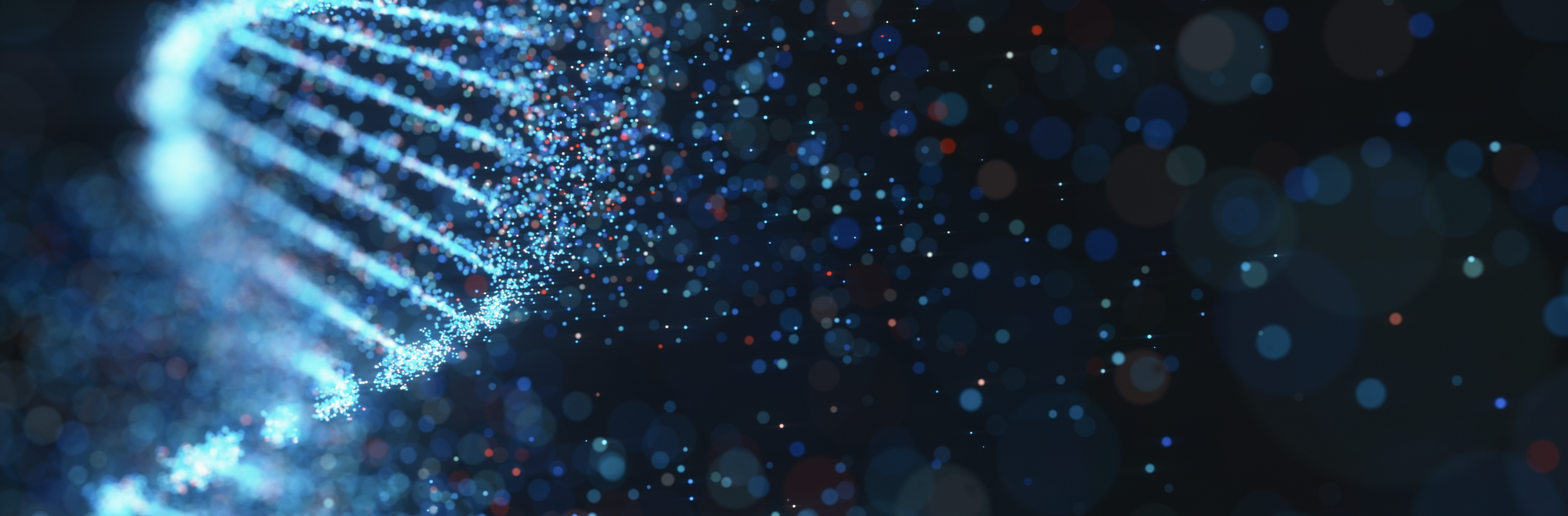
De Ridder Group
Bioinformatics
We are a bioinformatics lab that creates and applies innovative data science methods to advance our understanding of disease biology and molecular medicine with a focus on cancer research.
To achieve this, we build on the latest developments in machine learning and artificial intelligence and aim to adopt advances in these fields to achieve omics data analysis and data integration strategies. We therefore also heavily rely on high-performance computing and statistics.
Research
Omics Machine Learning with impact
We specialize in developing advanced machine learning and artificial intelligence algorithms to achieve various critical (pre-)clinical objectives using modern omics data. For instance, with Sturgeon we’ve cut down drastically on the time required for (pediatric) CNS cancer diagnostics with intraoperative native DNA Nanopore sequencing and methylation classification using deep learning. This is a clear example of our constant quest for actual, clinical, bedside impact. To facilitate these results, we also focus on laying the necessary groundwork, such as by rigorously comparing the newest Nanopore base-calling models and studying colorectal cancer cell evolution at a single-cell level. Interest areas in the lab are broad and range from GWAS, to liquid biopsy classification under data sparsity, to 3D genome conformation and epigenetics. Additionally, we devised approaches to identify robust gene-sets or pathways that differentiate between breast cancer subtypes or cancer treatment. In this endeavor, we employ machine learning models that can exploit existing biological knowledge, such as network- and pathway-based classifiers. In all these varied endeavors, translating from fundamental research to actual patient benefit is a guiding principle.
Next-generation diagnostics
We create classification models for patient cohort and clinical trial data to improve diagnostics and enable personalization of treatment strategies. Our track record includes the development of classifiers which can predict treatment benefit in multiple myeloma and colorectal cancer. The aforementioned liquid biopsy-based cancer type classification in sparse data conditions is only our first foray into the exciting new world of cell-free DNA diagnostics, which holds the promise to greatly increase patient comfort while at the same time enabling precision diagnoses. For the development of new liquid biopsy diagnostic applications, we harness state-of-the-art sequencing technologies. Notably, in collaboration with the Kloosterman lab, we have introduced CyclomicsSeq, a groundbreaking method for profiling cell-free tumor DNA through nanopore sequencing, which forms the foundation of our spin-out company, Cyclomics BV. Moreover, we are actively engaged in various projects focused on detecting base modifications and harnessing them for applications in cancer diagnostics.
Driving innovation through collaboration
We actively participated in a wide array of collaborative projects. For instance, we partnered with the van Oudenaarden lab to investigate cell lineage tracing in colon cancer organoid models, contributing to a deeper understanding of tumor evolution. Additionally, in collaboration with the de Laat lab, we co-developed Multi-Contact 4C, an innovative chromatin conformation capture strategy capable of identifying complex multi-way chromatin interactions using long-read sequencing. Currently, we are working together within an Oncode Accelerator consortium focused on ML applications for revolutionizing the development of cancer treatments, where we investigate the clinical translation of the wave of self-supervised foundation models.
Selected publications
Vermeulen C., Pagès-Gallego, M., Kester, L., Kranendonk, M.E.G., Wesseling, P., Verburg, N., de Witt Hamer, P., Kooi, E.J., Dankmeijer, L., van der Lugt, J., van Baarsen, K., Hoving, E.W., Tops, B.B.J. and de Ridder, J., 2023. Ultra-fast deep-learned CNS tumour classification during surgery. Nature.
Previously available as preprint on medRxiv.
Pagès-Gallego, M. and de Ridder, J., 2023. Comprehensive benchmark and architectural analysis of deep learning models for nanopore sequencing basecalling. Genome Biol.
Pedersen, B.S. and de Ridder, J., 2023. Echtvar: compressed variant representation for rapid annotation and filtering of SNPs and indels. Nucleic Acids Res.
Kester, L., de Barbanson, B., Lyubimova, A., Chen, L.T., van der Schrier, V., Alemany, A., Mooijman, D., Peterson-Maduro, J., Drost, J., de Ridder, J. and van Oudenaarden, A., 2022. Integration of multiple lineage measurements from the same cell reconstructs parallel tumor evolution. Cell Genomics, 2(2), p.100096.
von Berg, J., Ten Dam, M., van der Laan, S.W. and de Ridder, J., 2022. PolarMorphism enables discovery of shared genetic variants across multiple traits from GWAS summary statistics. Bioinformatics.
Danyi, A., Jager, M. and de Ridder, J., 2021 Cancer Type Classification in Liquid Biopsies Based on Sparse Mutational Profiles Enabled through Data Augmentation and Integration. Life (Basel).
Nieboer, M.M., Nguyen, L. and de Ridder, J., 2021. Predicting pathogenic non-coding SVs disrupting the 3D genome in 1646 whole cancer genomes using multiple instance learning. Scientific Reports, 11(1), pp.1-13.
Mulet-Lazaro, R., van Herk, S., Erpelinck, C., Bindels, E., Sanders, M.A., Vermeulen, C., Renkens, I., Valk, P., Melnick, A.M., de Ridder, J. and Rehli, M., 2021. Allele-specific expression of GATA2 due to epigenetic dysregulation in CEBPA double-mutant AML. Blood.
Marcozzi, A., Jager, M., Elferink, M., Straver, R., van Ginkel, J.H., Peltenburg, B., Chen, L.T., Renkens, I., van Kuik, J., Terhaard, C., de Bree, R., Devriese, L.A., Willems, S.M., Kloosterman, W.P., de Ridder, J., 2020. Accurate detection of circulating tumor DNA using nanopore consensus sequencing. npj Genom. Med. 6, 106.
Ubels, J., Schaefers, T., Punt, C., Guchelaar, H.J. and de Ridder, J., 2020. RAINFOREST: a random forest approach to predict treatment benefit in data from (failed) clinical drug trials. Bioinformatics, 36, pp.i601-i609.
Kuzniar, A., Maassen, J., Verhoeven, S., Santuari, L., Shneider, C., Kloosterman, W.P. and de Ridder, J., 2020. sv-callers: a highly portable parallel workflow for structural variant detection in whole-genome sequence data. PeerJ, 8, p.e8214.
Vermeulen, C., Allahyar, A., Bouwman, B.A., Krijger, P.H., Verstegen, M.J., Geeven, G., Valdes-Quezada, C., Renkens, I., Straver, R., Kloosterman, W.P. and de Ridder, J., 2020. Multi-contact 4C: long-molecule sequencing of complex proximity ligation products to uncover local cooperative and competitive chromatin topologies. Nature protocols, 15(2), pp.364-397.
Ubels, J., Sonneveld, P., van Vliet, M.H. and de Ridder, J., 2020. Gene networks constructed through simulated treatment learning can predict proteasome inhibitor benefit in multiple myeloma. Clinical Cancer Research, 26(22), pp.5952-5961.
Nieboer, M.M. and de Ridder, J., 2020. svMIL: predicting the pathogenic effect of TAD boundary-disrupting somatic structural variants through multiple instance learning. Bioinformatics, 36, pp.i692-i699.
Allahyar, A., Ubels, J. and de Ridder, J., 2019. A data-driven interactome of synergistic genes improves network-based cancer outcome prediction. PLoS computational biology, 15(2), p.e1006657.
Ubels, J., Sonneveld, P., van Beers, E.H., Broijl, A., van Vliet, M.H. and de Ridder, J., 2018. Predicting treatment benefit in multiple myeloma through simulation of alternative treatment effects. Nature communications, 9(1), pp.1-10.
Allahyar, A., Vermeulen, C., Bouwman, B.A., Krijger, P.H., Verstegen, M.J., Geeven, G., van Kranenburg, M., Pieterse, M., Straver, R., Haarhuis, J.H. and Jalink, K., 2018. Enhancer hubs and loop collisions identified from single-allele topologies. Nature genetics, 50(8), pp.1151-1160.
Allahyar, A. and De Ridder, J., 2015. FERAL: network-based classifier with application to breast cancer outcome prediction. Bioinformatics, 31(12), pp.i311-i319.
Group members
Jeroen de Ridder
Principal Investigator / Full Professor
Adrien Melquiond
Assistant Professor
Carlo Vermeulen
Assistant Professor
Lucía Barbadilla
PhD Student
Dieter Stoker
PhD Student
Huub van der Ent
PhD Student
Ahmadreza Iranpour
PhD Student
Carlos Garcia Fernandez
PhD Student
Cas Kranenburg
Research Technician
Li-Ting Chen
PhD Student
Emmy Wesdorp
PhD Student
Joanna Wolthuis
PhD Student
Guest researcher(s)
Marta Moreno Gonzalez
PhD Student
Merel Jongmans
PhD Student
Jack Jiang
PhD Student
Lab Alumni
Amin Allahyar
Buys de Barbanson
Tilman Schaefers
Jasmin Böhmer
Joanna von Berg
Joske Ubels
Marleen Nieboer
Stefania Magnusdottir
Joachim Kutzera
Brent Pederson
Luca Santuari
Nicolle Besselink
Marc Pagès-Gallego